Yeah, that’s about right:
Tag: board games
[Bloganuary] Playtime
This post is part of my attempt at Bloganuary 2024. Today’s prompt is:
Do you play in your daily life? What says “playtime” to you?
How do I play? Let me count the ways!
RPGs
I’m involved in no fewer than three different RPG campaigns (DMing the one for The Levellers) right now, plus periodic one-shots. I love a good roleplaying game, especially one that puts character-building and storytelling above rules-lawyering and munchkinery, specifically because that kind of collaborative, imaginative experience feels more like the kind of thing we call “play” when done it’s done by children!
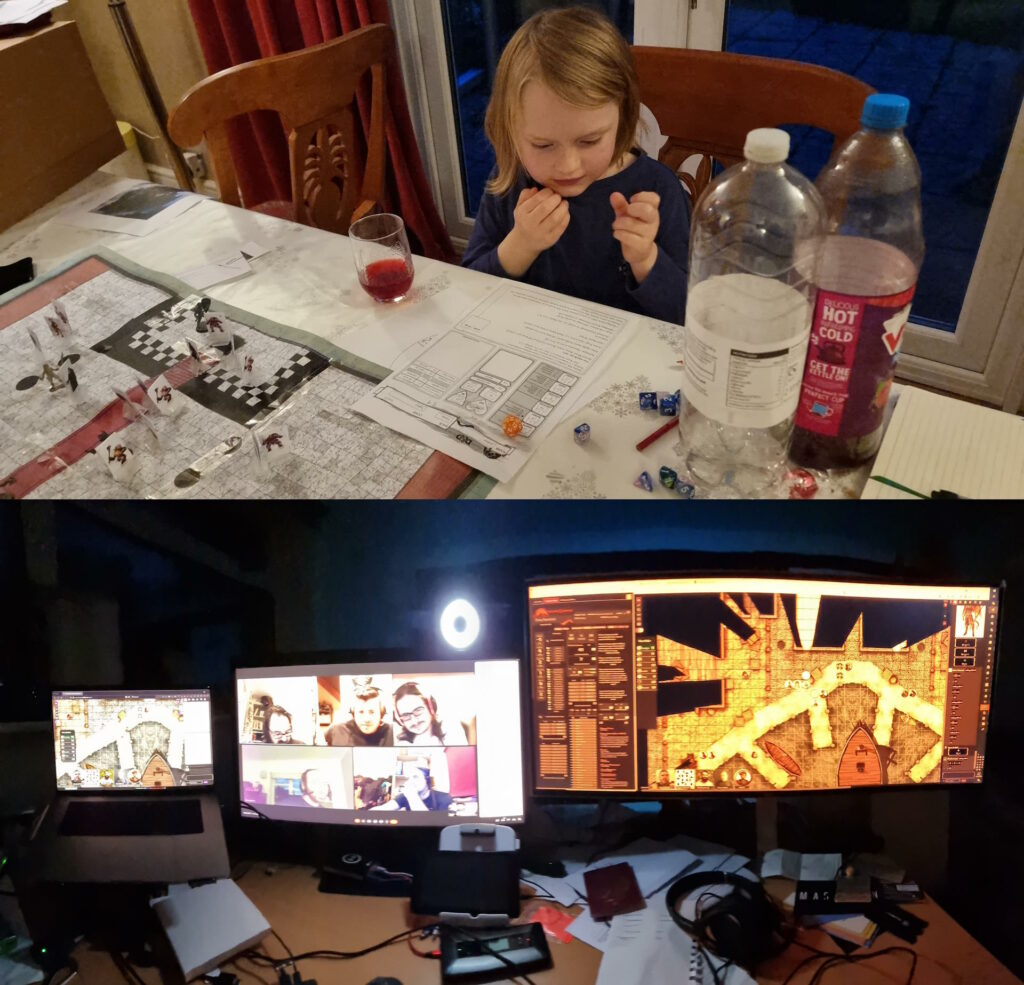
Videogames
I don’t feel like I get remotely as much videogaming time as I used to, and in theory I’ve become more-selective about exactly what I spend my time on1.
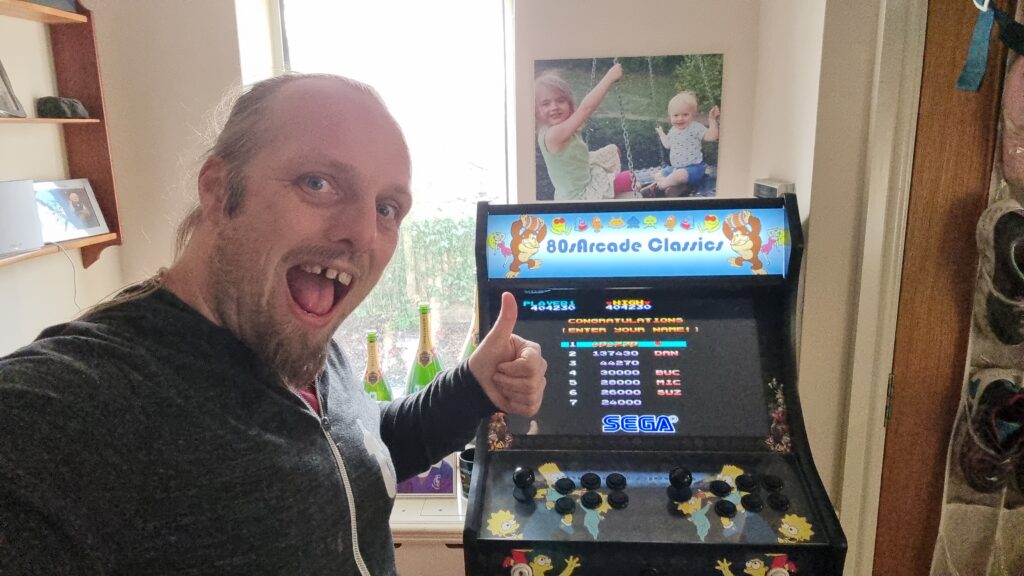
Board Games
Similarly, I don’t feel like I get as much time to grind through my oversized board games collection as I used to2, but that’s improving as the kids get older and can be roped-into a wider diversity of games3.
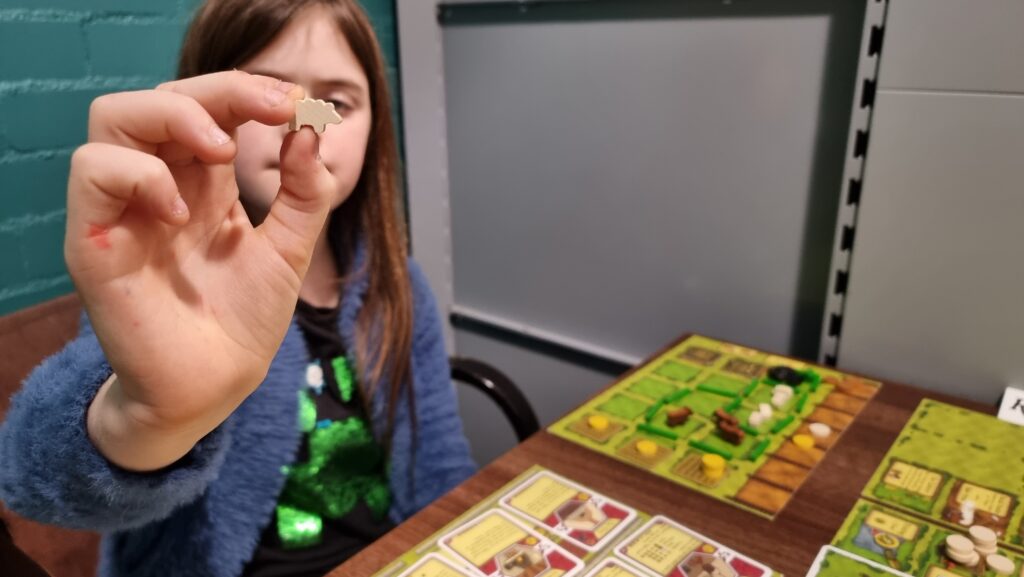
Escape Rooms
I love a good escape room, and I can’t wait until the kids are old enough for (more of) them too so I’ve an excuse to do more of them. When we’re not playing conventional escape rooms, Ruth and I can sometimes be found playing board game-style boxed “kit” ones (which have very variable quality, in my experience) and we’ve recently tried a little Escape Academy.
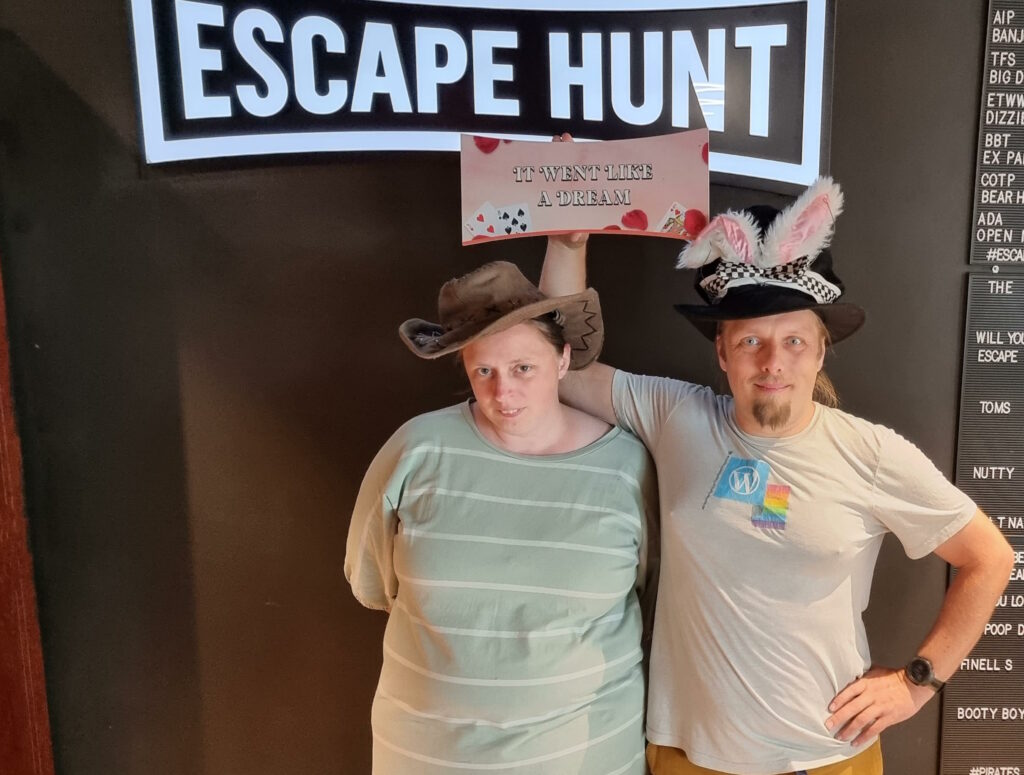
GNSS Activities
I’m sure everybody knows I do a modest amount of geocaching and geohashing.4
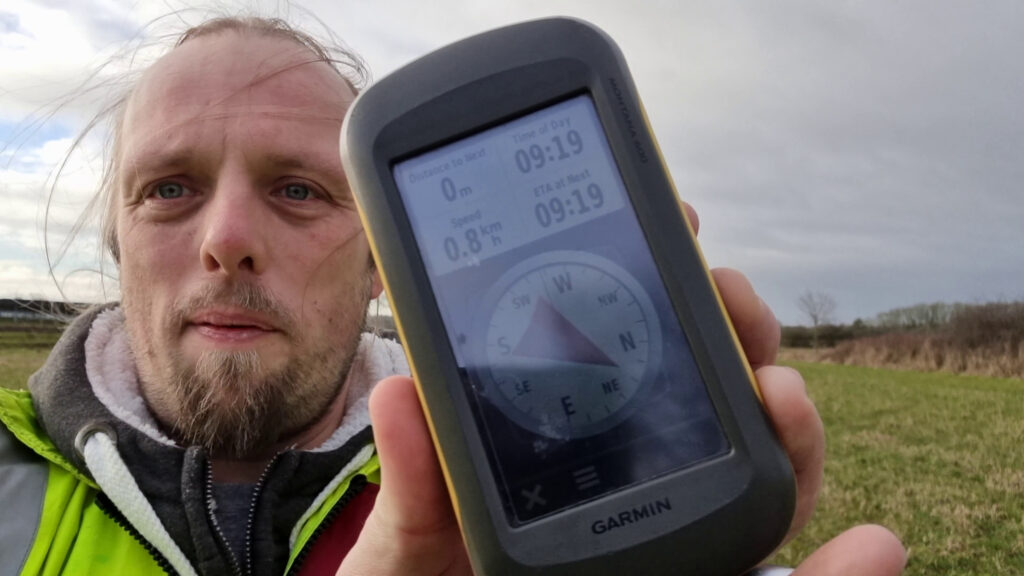
They’re not the only satnav-based activities I do at least partially “for fun” though! I contribute to OpenStreetMap, often through the “gamified” experience of the StreetComplete app, and I’m very slowly creeping up the leader board at OpenBenches. Are these “play”? Sure, maybe.
And all of the above is merely the structured kinds of play I engage in. Playing “let’s pretend”-style games with the kids (even when they make it really, really weird) adds a whole extra aspect. Also there’s the increasingly-rare murder mystery parties we sometimes hold: does that count as roleplaying, or some other kind of play?
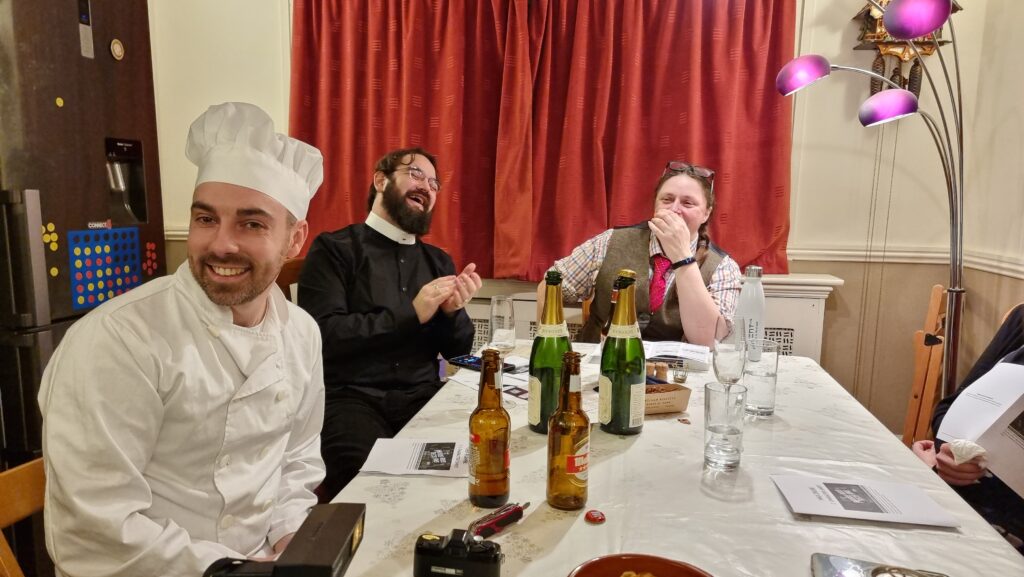
Suffice to say, there’s plenty of play in my life, it’s quite varied and diverse, and there is, if anything, not enough of it!
Footnotes
1 I say that, and yet somehow Steam tells me that one of my most-played games this year was Starfield, which was… meh? Apparently compelling enough that I’ve “ascended” twice, but in hindsight I wish I hadn’t bothered.
2 Someday my group and I will finish Pandemic Legacy: Season 2 so we can get started on Season 0 which has sat unplayed on my shelves since I got it… oooh… two or three years ago‽
3 This Christmas, I got each of them their first “legacy” game: Zombie Kids for the younger one, My City for the elder. They both seem pretty good.
4 Geocaching is where you use military satellite networks to find lost tupperware. Geohashing uses the same technology but what you find is a whole lot of nothing. I don’t think I can explain why I find the latter more-compelling.
Paul Labyrinth
This article is a repost promoting content originally published elsewhere. See more things Dan's reposted.
Known Leaders is an open-source program that combines WikiData with a random generator to come up with almost-invariably inaccurate but sometimes hilarious facts. Jim Kang came up with it during Recurse Center‘s Never Graduate Week. Go have a play, or read more about how and why he made it.
Have Fun with Missions, Visions, and Values
I just spent a lightweight week in Rome with fellow members of Automattic‘s Team Fire.
Among our goals for the week was an attempt to strengthen the definition of who are team are, what we work on, and how and why we do so. That’s basically a team-level identity, mission, vision, and values, right?
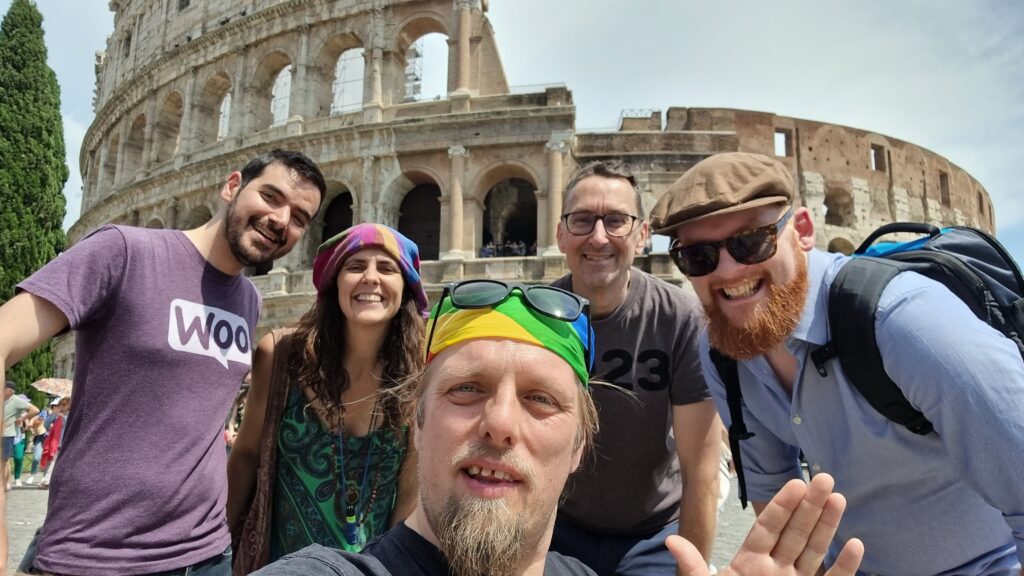
Fellow Automattician Ben Dwyer recently wrote about his experience of using a deck of Dixit cards to help his team refine their values in a fun and engaging way. I own a Dixit set, so we decided to give it a go too.
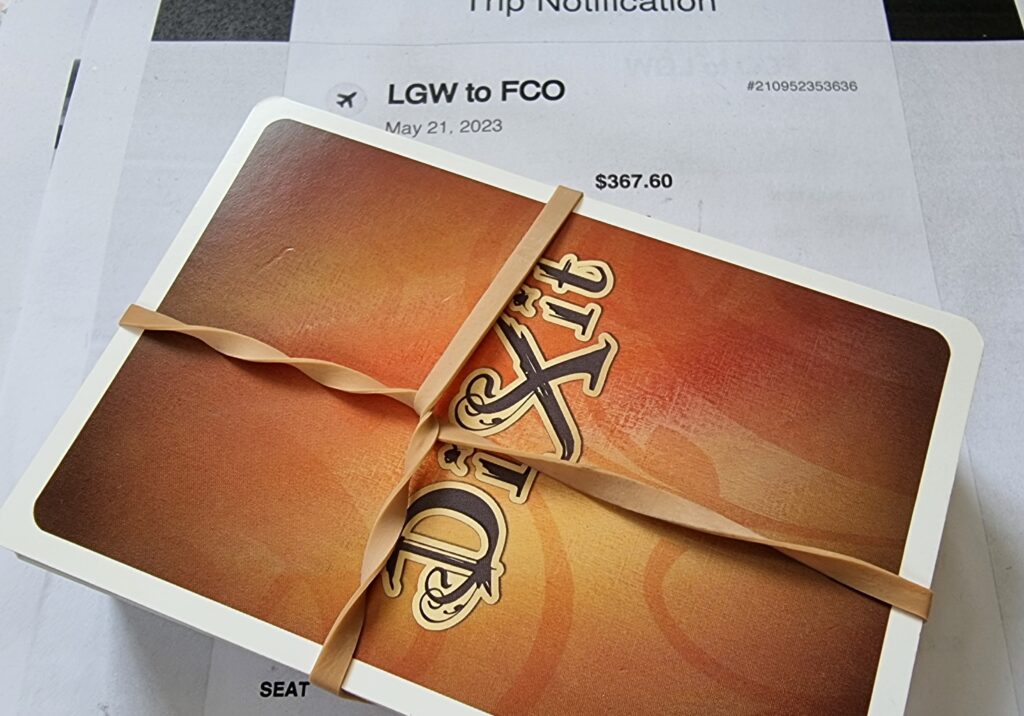
Normally when you play Dixit, you select a card from your hand – each shows a unique piece of artwork – and try to describe it in a way that’s precise enough that some of the other players will later be able to pick it out of a line-up, but ambiguous enough that not all the other players will. It’s a delicate balancing act. Even when our old Geek Night was in full swing we didn’t used to play it often because our well-established group’s cornucopia of in-jokes and references made it trivially easy to “target” your descriptions at specific players1, but it’s still a solid icebreaker activity.
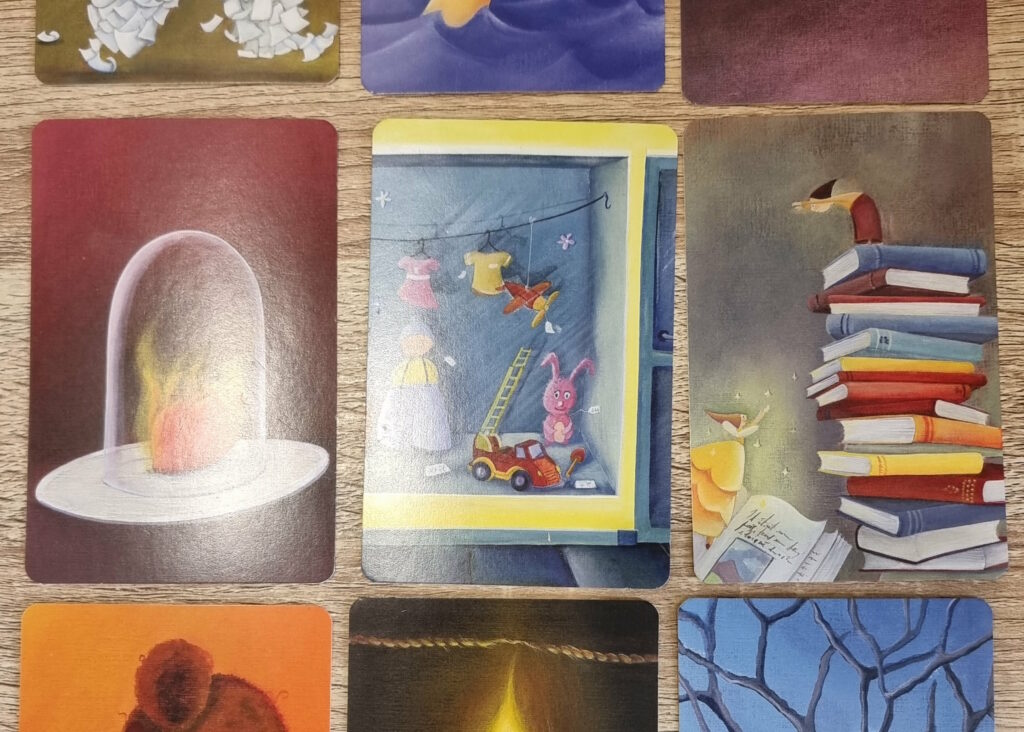
Perhaps it was the fantasy artwork that inspired us or maybe it just says something about how my team sees themselves, but what we came up with had a certain… swords-and-sorcery… even Dungeons & Dragons… feel to it.
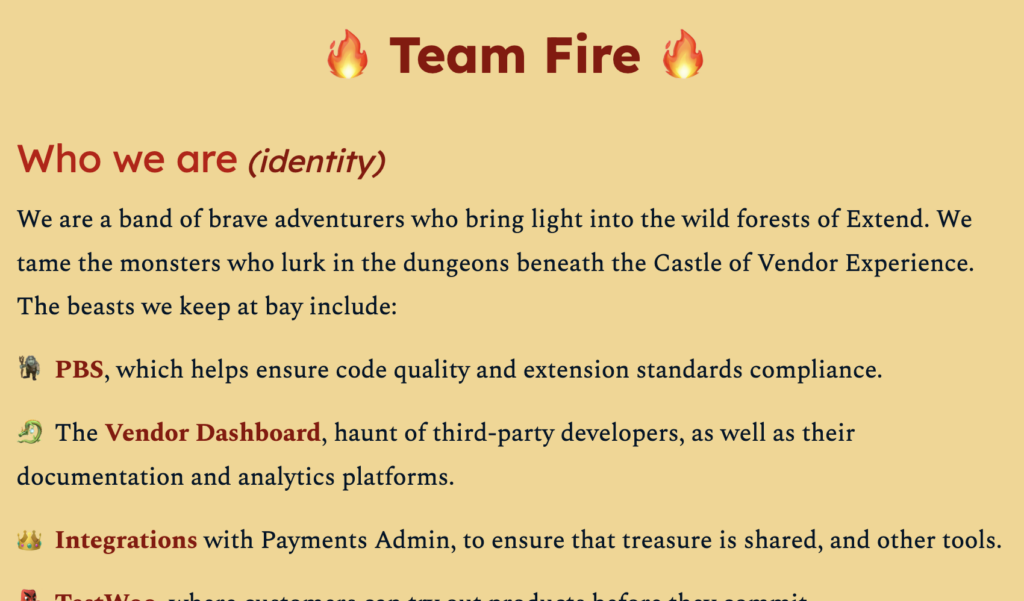
Ou team’s new identity isn’t finalised, but I love the fact that we’ve been able to inject a bit of fun and whimsy into it. At our last draft, my team looks to be defined as comprising:
- Gareth, level 62 Pathfinder, leading the way through the wilds
- Bero, Level 5 Battlesmith, currently lost in the void
- Dan (me!), Level 5 Arcane Trickster, breaking locks and stealing treasure
- Cem, Level 4 Dragonslayer, smashing doors and bugs alike
- Lae, Level 7 Pirate, seabound rogue with eyes on the horizon
- Kyle, Level 5 Apprentice Bard, master of words and magic
- Simran, Level 6 Apprentice Code Witch, weaving spells from nature
I think that’s pretty awesome.
Sisyphus: The Board Game (Digital Edition)
I’m off work sick today: it’s just a cold, but it’s had a damn good go at wrecking my lungs and I feel pretty lousy. You know how when you’ve got too much of a brain-fog to trust yourself with production systems but you still want to write code (or is that just me?), so this morning I threw together a really, really stupid project which you can play online here.
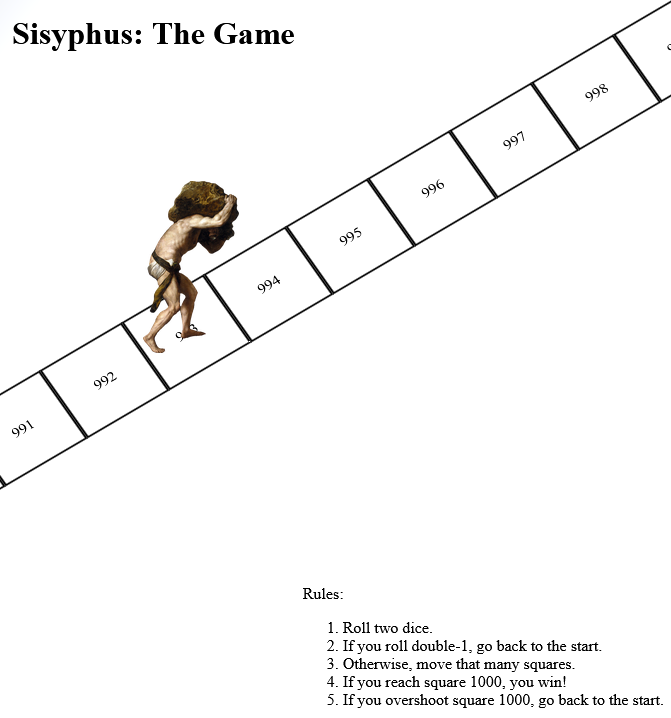
It’s inspired by a toot by Mason”Tailsteak” Williams (whom I’ve mentioned before once or twice). At first I thought I’d try to calculate the odds of winning at his proposed game, or how many times one might expect to play before winning, but I haven’t the brainpower for that in my snot-addled brain. So instead I threw together a terrible, terrible digital implementation.
Go play it if, like me, you’ve got nothing smarter that your brain can be doing today.
Beating Children at Mastermind [Video]
This video accompanies a blog post of the same title. The content is basically the same – if you prefer videos, watch this video. If you prefer blog posts, go read the blog post. You might also like to play with my Mastermind solver or view the source code.
Beating Children at Mastermind
This blog post is also available as a video. Would you prefer to watch/listen to me tell you about how I’ve implemented a tool to help me beat the kids when we play Mastermind?
I swear that I used to be good at Mastermind when I was a kid. But now, when it’s my turn to break the code that one of our kids has chosen, I fail more often than I succeed. That’s no good!
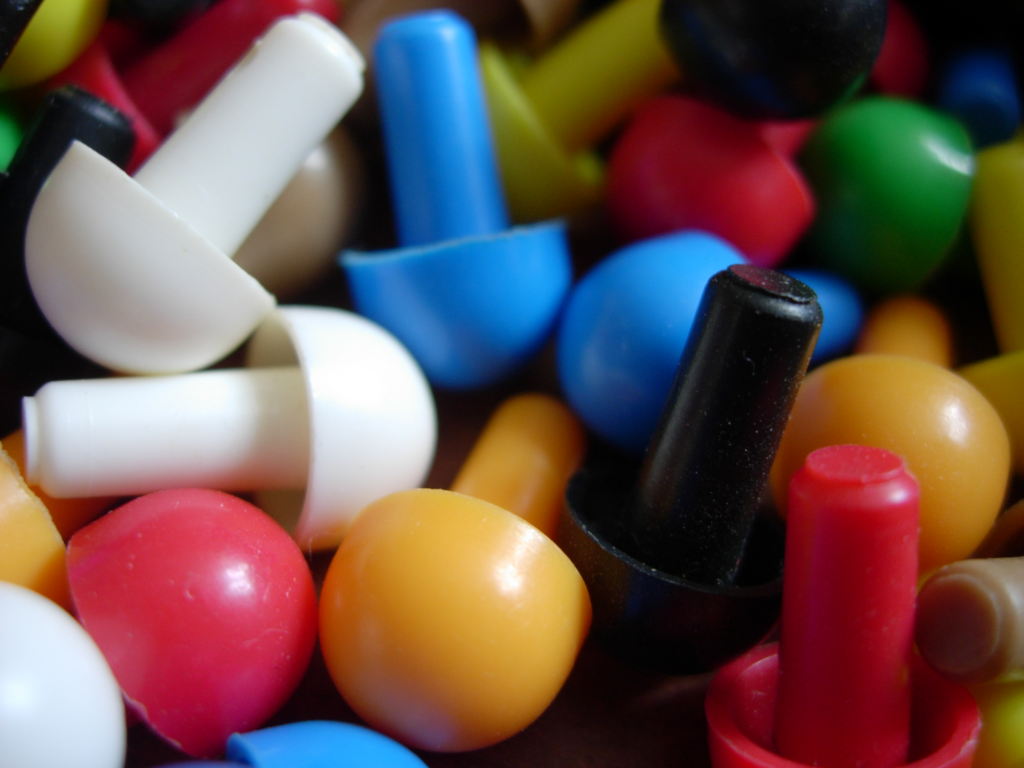
Mastermind and me
Maybe it’s because I’m distracted; multitasking doesn’t help problem-solving. Or it’s because we’re “Super” Mastermind, which differs from the one I had as a child in that eight (not six) peg colours are available and secret codes are permitted to have duplicate peg colours. These changes increase the possible permutations from 360 to 4,096, but the number of guesses allowed only goes up from 8 to 10. That’s hard.
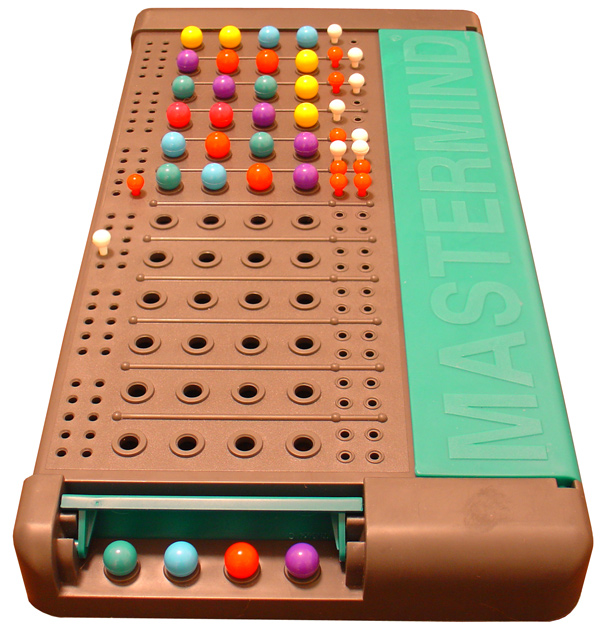
Or maybe it’s just that I’ve gotten lazy and I’m now more-likely to try to “solve” a puzzle using a computer to try to crack a code using my brain alone. See for example my efforts to determine the hardest hangman words and make an adverserial hangman game, to generate solvable puzzles for my lock puzzle game, to cheat at online jigsaws, or to balance my D&D-themed Wordle clone.
Hey, that’s an idea. Let’s crack the code… by writing some code!
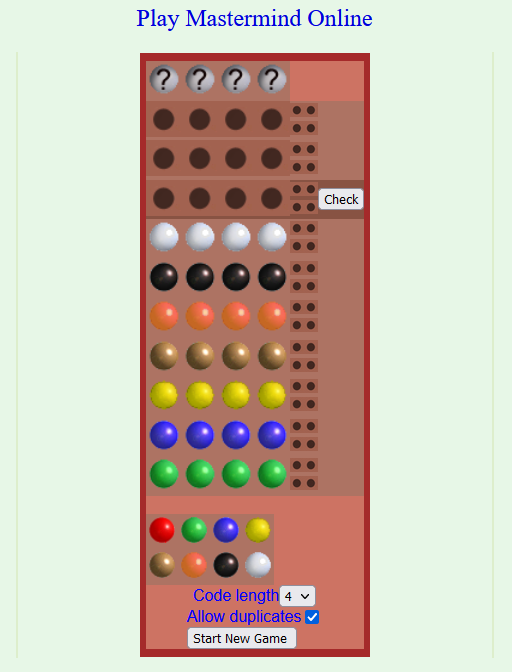
Representing a search space
The search space for Super Mastermind isn’t enormous, and it lends itself to some highly-efficient computerised storage.
There are 8 different colours of peg. We can express these colours as a number between 0 and 7, in three bits of binary, like this:
Decimal | Binary | Colour |
---|---|---|
0
|
000
|
Red |
1
|
001
|
Orange |
2
|
010
|
Yellow |
3
|
011
|
Green |
4
|
100
|
Blue |
5
|
101
|
Pink |
6
|
110
|
Purple |
7
|
111
|
White |
There are four pegs in a row, so we can express any given combination of coloured pegs as a 12-bit binary number. E.g. 100 110 111 010
would represent the
permutation blue (100
), purple (110
), white (111
), yellow (010
). The total search space, therefore, is the range of numbers from
000000000000
through 111111111111
… that is: decimal 0 through 4,095:
Decimal | Binary | Colours |
---|---|---|
0
|
000000000000
|
Red, red, red, red |
1
|
000000000001
|
Red, red, red, orange |
2
|
000000000010
|
Red, red, red, yellow |
………… | ||
4092
|
|
White, white, white, blue |
4093
|
|
White, white, white, pink |
4094
|
|
White, white, white, purple |
4095
|
|
White, white, white, white |
Whenever we make a guess, we get feedback in the form of two variables: each peg that is in the right place is a bull; each that represents a peg in the secret code but isn’t in the right place is a cow (the names come from Mastermind’s precursor, Bulls & Cows). Four bulls would be an immediate win (lucky!), any other combination of bulls and cows is still valuable information. Even a zero-score guess is valuable- potentially very valuable! – because it tells the player that none of the pegs they’ve guessed appear in the secret code.
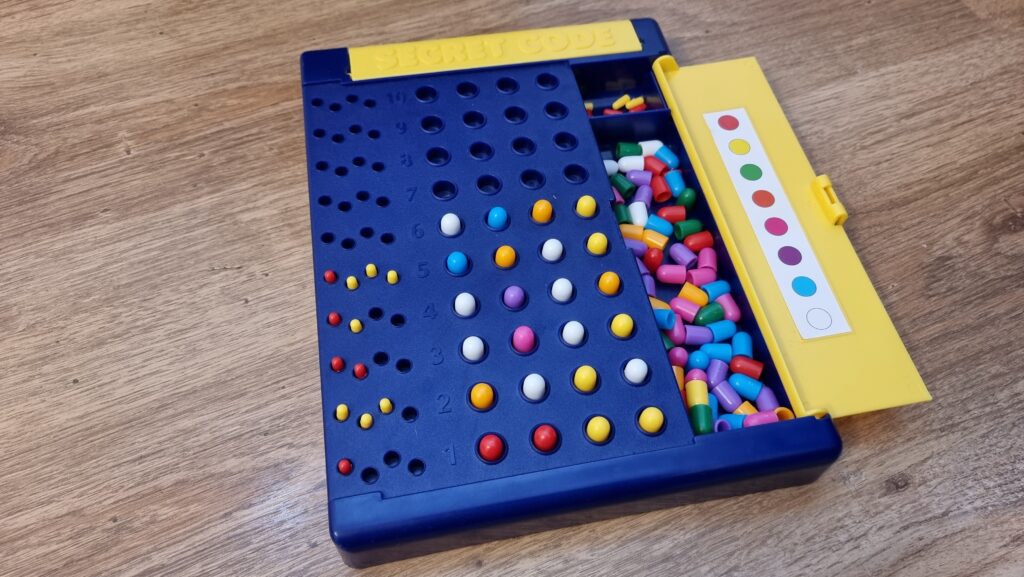
Solving with Javascript
The latest versions of Javascript support binary literals and bitwise operations, so we can encode and decode between arrays of four coloured pegs (numbers 0-7) and the number 0-4,095
representing the guess as shown below. Decoding uses an AND bitmask to filter to the requisite digits then divides by the order of magnitude. Encoding is just a reduce
function that bitshift-concatenates the numbers together.
116 117 118 119 120 121 122 123 124 125 126 127 128 129 130 131 132 133 134 |
/** * Decode a candidate into four peg values by using binary bitwise operations. */ function decodeCandidate(candidate){ return [ (candidate & 0b111000000000) / 0b001000000000, (candidate & 0b000111000000) / 0b000001000000, (candidate & 0b000000111000) / 0b000000001000, (candidate & 0b000000000111) / 0b000000000001 ]; } /** * Given an array of four integers (0-7) to represent the pegs, in order, returns a single-number * candidate representation. */ function encodeCandidate(pegs) { return pegs.reduce((a, b)=>(a << 3) + b); } |
With this, we can simply:
- Produce a list of candidate solutions (an array containing numbers 0 through 4,095).
- Choose one candidate, use it as a guess, and ask the code-maker how it scores.
- Eliminate from the candidate solutions list all solutions that would not score the same number of bulls and cows for the guess that was made.
- Repeat from step #2 until you win.
Step 3’s the most important one there. Given a function getScore( solution, guess )
which returns an array of [ bulls, cows ]
a given guess
would
score if faced with a specific solution
, that code would look like this (I’m convined there must be a more-performant way to eliminate candidates from the list with XOR
bitmasks, but I haven’t worked out what it is yet):
164 165 166 167 168 169 170 171 172 173 174 175 176 177 178 179 180 181 182 |
/** * Given a guess (array of four integers from 0-7 to represent the pegs, in order) and the number * of bulls (number of pegs in the guess that are in the right place) and cows (number of pegs in the * guess that are correct but in the wrong place), eliminates from the candidates array all guesses * invalidated by this result. Return true if successful, false otherwise. */ function eliminateCandidates(guess, bulls, cows){ const newCandidatesList = data.candidates.filter(candidate=>{ const score = getScore(candidate, guess); return (score[0] == bulls) && (score[1] == cows); }); if(newCandidatesList.length == 0) { alert('That response would reduce the candidate list to zero.'); return false; } data.candidates = newCandidatesList; chooseNextGuess(); return true; } |
I continued in this fashion to write a full solution (source code). It uses ReefJS for component rendering and state management, and you can try it for yourself right in your web browser. If you play against the online version I mentioned you’ll need to transpose the colours in your head: the physical version I play with the kids has pink and purple pegs, but the online one replaces these with brown and black.
Testing the solution
Let’s try it out against the online version:
As expected, my code works well-enough to win the game every time I’ve tried, both against computerised and in-person opponents. So – unless you’ve been actively thinking about the specifics of the algorithm I’ve employed – it might surprise you to discover that… my solution is very-much a suboptimal one!
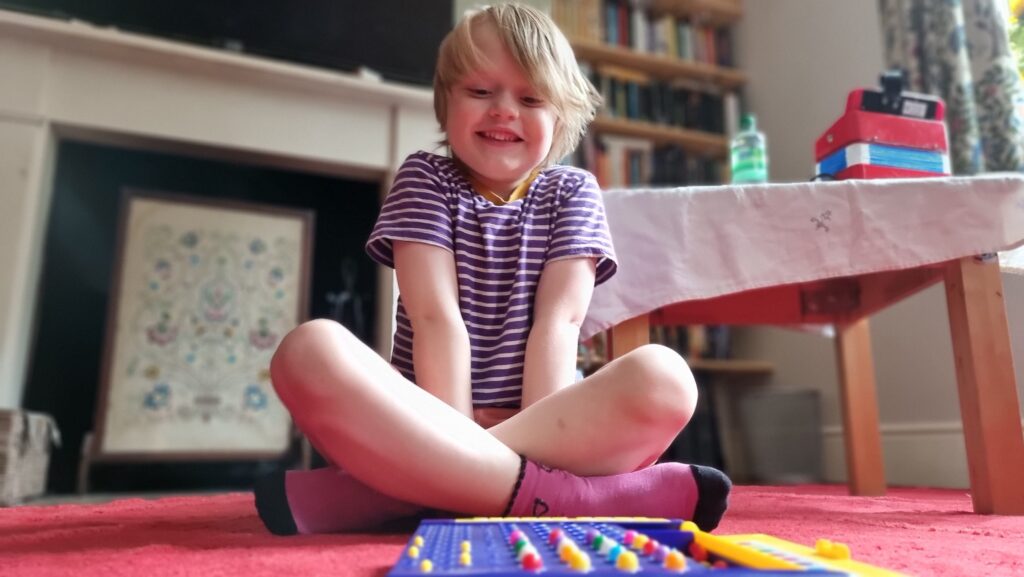
My solution is suboptimal
A couple of games in, the suboptimality of my solution became pretty visible. Sure, it still won every game, but it was a blunt instrument, and anybody who’s seriously thought about games like this can tell you why. You know how when you play e.g. Wordle (but not in “hard mode”) you sometimes want to type in a word that can’t possibly be the solution because it’s the best way to rule in (or out) certain key letters? This kind of strategic search space bisection reduces the mean number of guesses you need to solve the puzzle, and the same’s true in Mastermind. But because my solver will only propose guesses from the list of candidate solutions, it can’t make this kind of improvement.
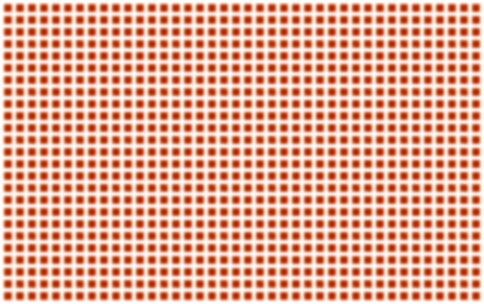
Search space bisection is also used in my adverserial hangman game, but in this case the aim is to split the search space in such a way that no matter what guess a player makes, they always find themselves in the larger remaining portion of the search space, to maximise the number of guesses they have to make. Y’know, because it’s evil.

There are mathematically-derived heuristics to optimise Mastermind strategy. The first of these came from none other than Donald Knuth (legend of computer science, mathematics, and pipe organs) back in 1977. His solution, published at probably the height of the game’s popularity in the amazingly-named Journal of Recreational Mathematics, guarantees a solution to the six-colour version of the game within five guesses. Ville [2013] solved an optimal solution for a seven-colour variant, but demonstrated how rapidly the tree of possible moves grows and the need for early pruning – even with powerful modern computers – to conserve memory. It’s a very enjoyable and readable paper.
But for my purposes, it’s unnecessary. My solver routinely wins within six, maybe seven guesses, and by nonchalantly glancing at my phone in-between my guesses I can now reliably guess our children’s codes quickly and easily. In the end, that’s what this was all about.
Printing Maps from Dungeondraft
I really love Dungeondraft, an RPG battle map generator. It’s got great compatibility with online platforms like Foundry VTT and Roll20, but if you’re looking to make maps for tabletop play, there’s a few tips I can share:
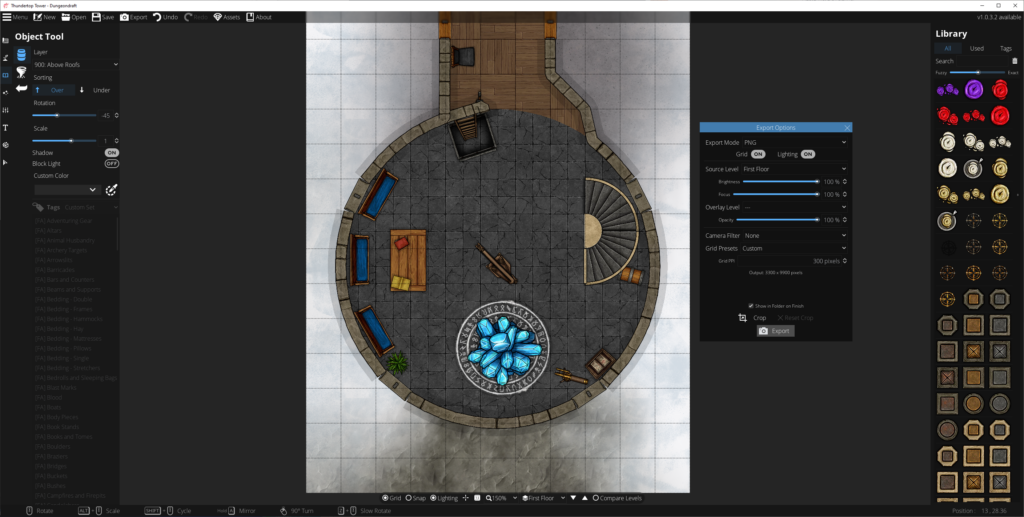
Planning and designing
Dungeondraft has (or can be extended with) features to support light levels and shadow-casting obstructions, openable doors and windows, line-of sight etc… great to have when you’re building for Internet-enabled tabletops, but pointless when you’re planning to print out your map! Instead:
- Think about scale: I’m printing to A4 sheets and using inch-size squares, so every 11 x 8 squares equates to one sheet of paper. Knowing this, I can multiply-up to a whole number of sheets of paper and this informs my decisions about how to best make use of the maps (and what will and won’t fit on my dining table!).
- Focus on legibility: Your printer probably won’t have the same kind of resolution as your screen, and your players can’t “zoom in” to get details. Play with the grid styles (under Map Settings) to find what works best for you, and try not to clash with your floor patterns. If you’re printing in monochrome, use the “Printer-Friendly” camera filter (also under Map Settings, or in the Export Options dialog) to convert to gorgeous line-art. Make sure critical elements have sufficient contrast that they’ll stand out when printed or your players might walk right over that chest, campfire, or bookshelf.
- Think about exposure: You don’t get digital “fog of war” on the tabletop! Think about how you’re going to reveal the map to your players: plan to print in multiple sections to put together, jigsaw style, or have card to “cover” bits of the map. Think about how the tool can help you here: e.g. if you’ve got multiple buildings the players can explore, use a higher “level” or roof layer to put roofs on your buildings, then print the relevant parts of that level separately: now you’ve got a thematic cover-up that you can remove to show the insides of the building. Go the other way around for secret doors: print the empty wall on your main map (so players can’t infer the location of the secret door by the inclusion of a cover-up) and the secret door/passage on the overlay, so you can stick it onto the map when they find it.
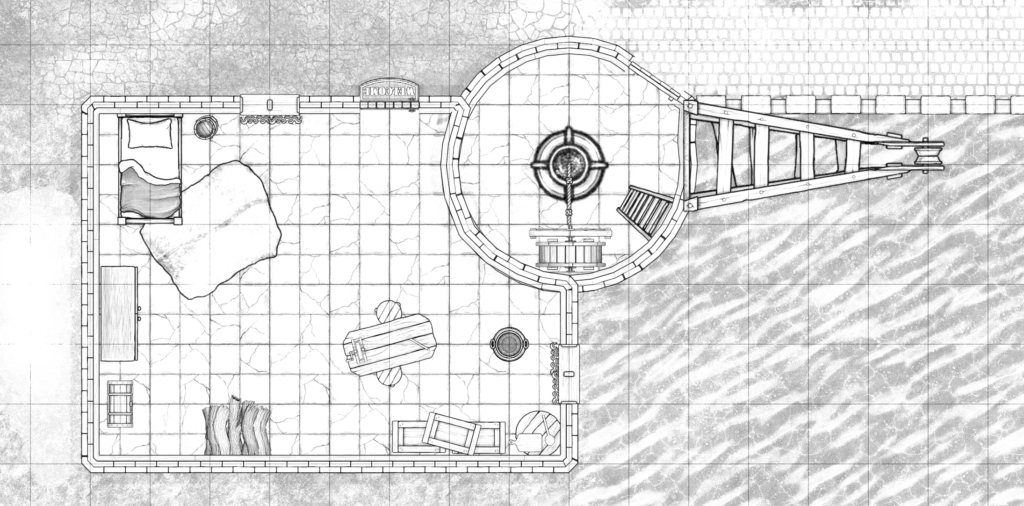
Printing it out
There’s no “print” option in Dungeondraft, so – especially if your map spans multiple “pages” – you’ll need a multi-step process to printing it out. With a little practice, it’s not too hard or time-consuming, though:
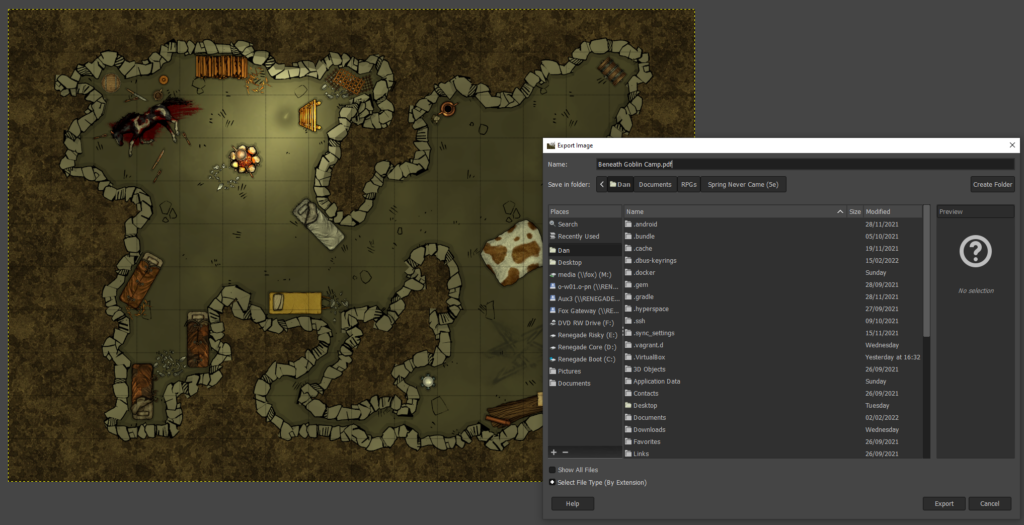
Export your map (level by level) from Dungeondraft as PNG files. The default settings are fine, but pay attention to the “Overlay level” setting if you’re using smart or complex cover-ups as described above.
To easily spread your map across multiple pages, you’ll need to convert it to a PDF. I’m using Gimp to do this. Simply open the PNG in Gimp, make any post-processing/last minute changes that you couldn’t manage in Dungeondraft, then click File >
Export As… and change the filename to have a .pdf
extension. You could print directly from Gimp, but in my experience PDF reader software does a much better job at multi-page printing.
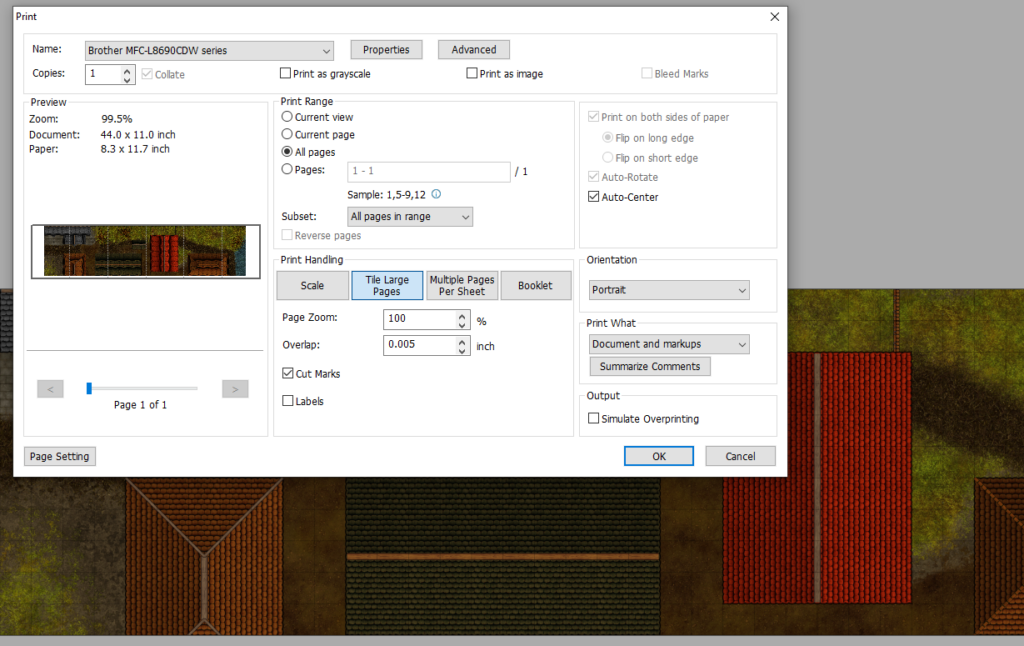
Open your PDF in an appropriate reader application with good print management. I’m using Foxit, which is… okay? Print it, selecting “tile large pages” to tell it to print across multiple sheets. Assuming you’ve produced a map an appropriate size for your printer’s margins, your preview should be perfect. If not, you can get away with reducing the zoom level by up to a percent or two without causing trouble for your miniatures. If you’d like the page breaks to occur at specific places (for exposure/reveal reasons), go back to Gimp and pad one side of the image by increasing the canvas size.
Check the level of “overlap” specified: I like to keep mine low and use the print margins as the overlapping part of my maps when I tape them together, but you’ll want to see how your printer behaves and adapt accordingly.
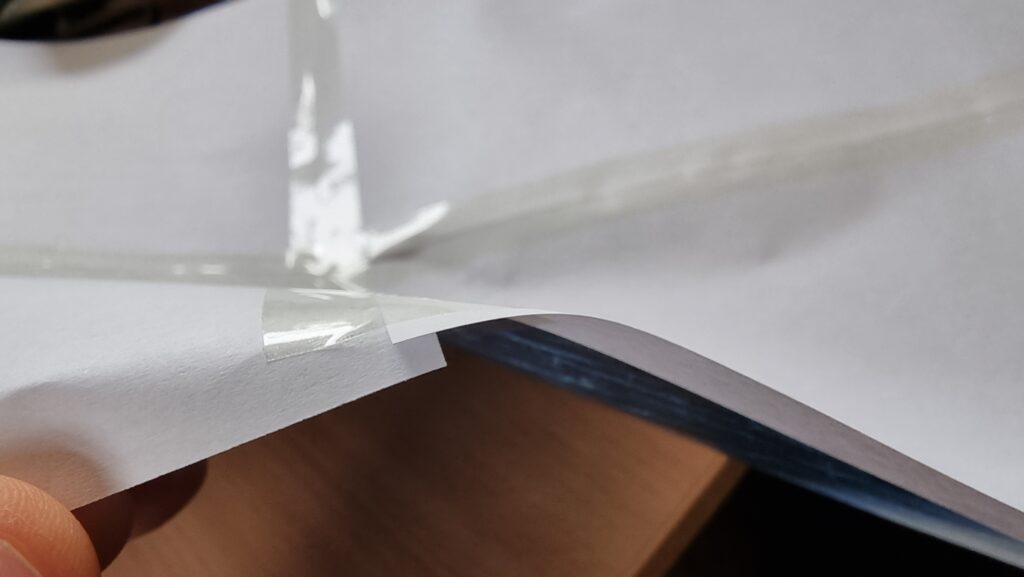
If you’re sticking together multiple pages to make a single large map, trim off the bottom and right margins of each page: if you printed with cut marks, this is easy enough even without a guillotine. Then tape them together on the underside, taking care to line-up the features on the map (it’s not just your players who’ll appreciate a good, visible grid: it’s useful when lining-up your printouts to stick, too!).
I keep my maps rolled-up in a box. If you do this too, just be ready with some paperweights to keep the edges from curling when you unfurl them across your gaming table. Or cut into separate rooms and mount to stiff card for that “jigsaw” effect! Whatever works best for you!
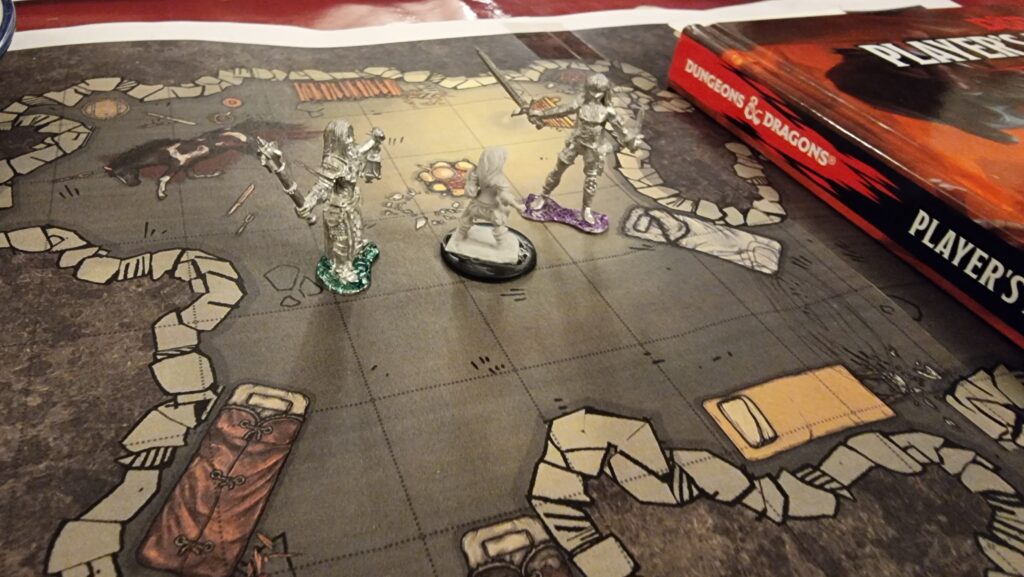
The Board Game Remix Kit
This article is a repost promoting content originally published elsewhere. See more things Dan's reposted.
New rules for old games! The Board Game Remix Kit is a collection of tips, tweaks, reimaginings and completely new games that you can play with the board and pieces from games you might already own: Monopoly, Cluedo, Scrabble and Trivial Pursuit.
The 26 rule tweaks and new games include:
- Full Houses: poker, but played with Monopoly properties
- Citygrid: a single-player city-building game
- Use Your Words: Scrabble with storytelling
- Them’s Fightin’ Words: a game of making anagrams, and arguing about which one would win in a fight
- Hunt the Lead Piping: hiding and searching for the Cluedo pieces in your actual house
- Guess Who Done It: A series of yes/no questions to identify the murderer (contributed by Meg Pickard)
- Zombie Mansion: use the lead piping to defend the Cluedo mansion
- Judy Garland on the Moon with a Bassoon: a drawing game that uses the answers to trivia questions as prompts
The Board Game Remix Kit was originally released in 2010 by the company Hide&Seek (which closed in 2014). We are releasing it here as a pdf (for phones/computers) and an epub (for ereaders) under a CC-BY-SA license.
If you enjoy the Kit and can afford it, please consider a donation to the World Health Organisation’s COVID-19 Response Fund.
Confined to your house? What a great opportunity to play board games with your fellow confinees.
Only got old family classics like Monopoly, Cluedo and Scrabble? Here’s a guide to mixing-them-up into new, fun, and highly-playable alternatives. Monopoly certainly needs it.
Cheating Hangman
A long while ago, inspired by Nick Berry‘s analysis of optimal Hangman strategy, I worked it backwards to find the hardest words to guess when playing Hangman. This week, I showed these to my colleague Grace – who turns out to be a fan of word puzzles – and our conversation inspired me to go a little deeper. Is it possible, I thought, for me to make a Hangman game that cheats by changing the word it’s thinking of based on the guesses you make in order to make it as difficult as possible for you to win?
The principle is this: every time the player picks a letter, but before declaring whether or not it’s found in the word –
- Make a list of all possible words that would fit into the boxes from the current game state.
- If there are lots of them, still, that’s fine: let the player’s guess go ahead.
- But if the player’s managing to narrow down the possibilities, attempt to change the word that they’re trying to guess! The new word must be:
- Legitimate: it must still be the same length, have correctly-guessed letters in the same places, and contain no letters that have been declared to be incorrect guesses.
- Harder: after resolving the player’s current guess, the number of possible words must be larger than the number of possible words that would have resulted otherwise.
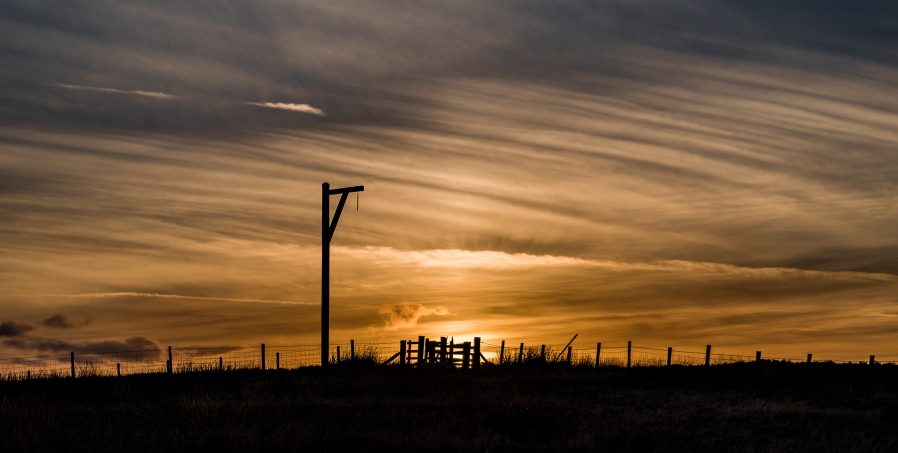
You might think that this strategy would just involve changing the target word so that you can say “nope” to the player’s current guess. That happens a lot, but it’s not always the case: sometimes, it’ll mean changing to a different word in which the guessed letter also appears. Occasionally, it can even involve changing from a word in which the guessed letter didn’t appear to one in which it does: that is, giving the player a “freebie”. This may seem counterintuitive as a strategy, but it sometimes makes sense: if saying “yeah, there’s an E at the end” increases the number of possible words that it might be compared to saying “no, there are no Es” then this is the right move for a cheating hangman.
Playing against a cheating hangman also lends itself to devising new strategies as a player, too, although I haven’t yet looked deeply into this. But logically, it seems that the optimal strategy against a cheating hangman might involve making guesses that force the hangman to bisect the search space: knowing that they’re always going to adapt towards the largest set of candidate words, a perfect player might be able to make guesses to narrow down the possibilities as fast as possible, early on, only making guesses that they actually expect to be in the word later (before their guess limit runs out!).
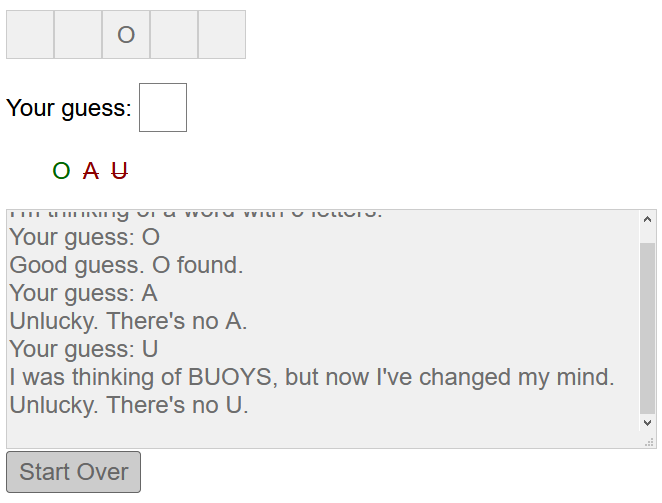
I also find myself wondering how easily I could adapt this into a “helpful hangman”: a game which would always change the word that you’re trying to guess in order to try to make you win. This raises the possibility of a whole new game, “suicide hangman”, in which the player is trying to get themselves killed and so is trying to pick letters that can’t possibly be in the word and the hangman is trying to pick words in which those letters can be found, except where doing so makes it obvious which letters the player must avoid next. Maybe another day.
In the meantime, you’re welcome to go play the game (and let me know what you think, below!) and, if you’re of such an inclination, read the source code. I’ve used some seriously ugly techniques to make this work, including regular expression metaprogramming (using regular expressions to write regular expressions), but the code should broadly make sense if you want to adapt it. Have fun!
Update 26 September 2019, 16:23: I’ve now added “helpful mode”, where the computer tries to cheat on your behalf rather than against you, but it’s not as helpful as you’d think because it assumes you’re playing optimally and have already memorised the dictionary!
Update 1 October 2019, 06:40: Now featured on MetaFilter; hi, MeFites!
What can board game strategy tell us about the future of the car wash?
I’m increasingly convinced that Friedemann Friese‘s 2009 board game Power Grid: Factory Manager (BoardGameGeek) presents gamers with a highly-digestible model of the energy economy in a capitalist society. In Factory Manager, players aim to financially-optimise a factory over time, growing production and delivery capacity through upgrades in workflow, space, energy, and staff efficiency. An essential driving factor in the game is that energy costs will rise sharply throughout. Although it’s not always clear in advance when or by how much, this increase in the cost of energy is always at the forefront of the savvy player’s mind as it’s one of the biggest factors that will ultimately impact their profit.
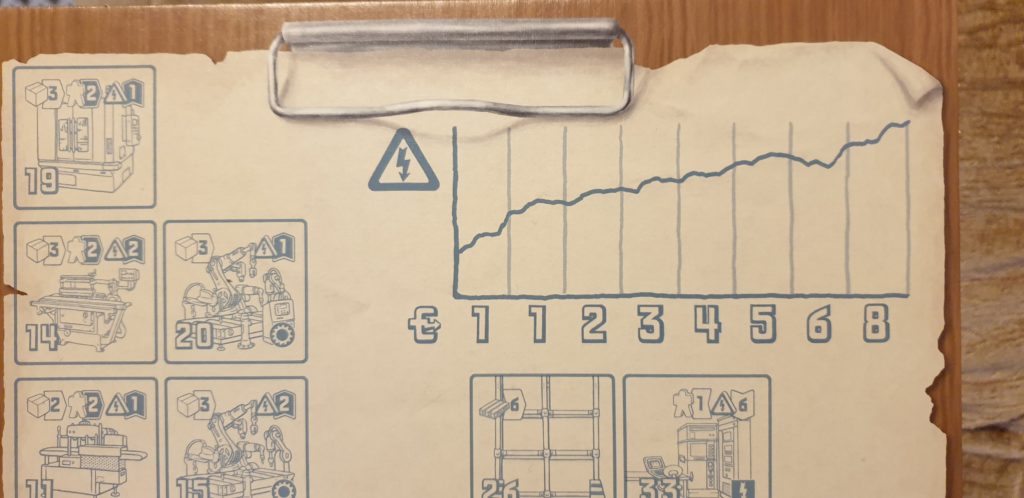
Given that players aim to optimise for turnover towards the end of the game (and as a secondary goal, for the tie-breaker: at a specific point five rounds after the game begins) and not for business sustainability, the game perhaps-accidentally reasonably-well represents the idea of “flipping” a business for a profit. Like many business-themed games, it favours capitalism… which makes sense – money is an obvious and quantifiable way to keep score in a board game! – but it still bears repeating.
There’s one further mechanic in Factory Manager that needs to be understood: a player’s ability to control the order in which they take their turn and their capacity to participate in the equipment auctions that take place at the start of each round is determined by their manpower-efficiency in the previous round. That is: a player who operates a highly-automated factory running on a skeleton staff benefits from being in the strongest position for determining turn order and auctions in their next turn.
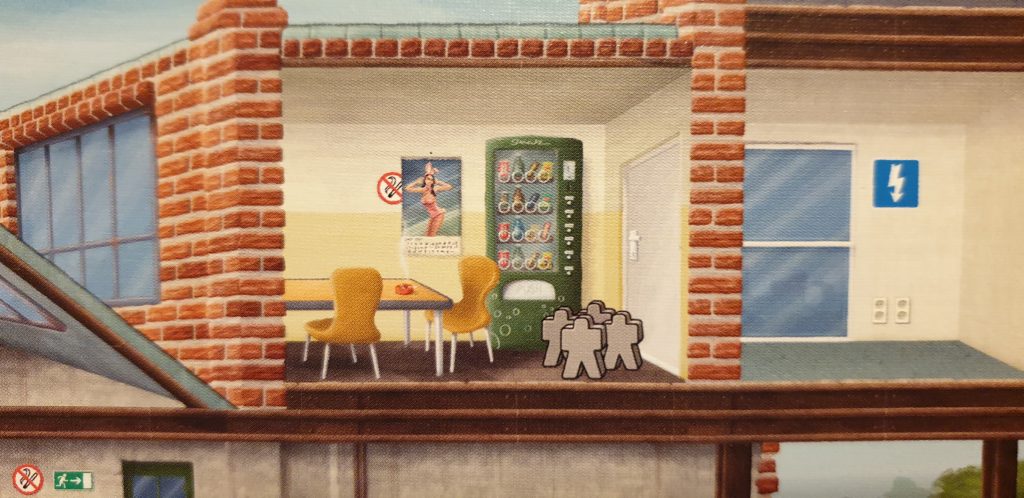
The combination of these rules leads to an interesting twist: in the final turn – when energy costs are at their highest and there’s no benefit to holding-back staff to monopolise the auction phase in the nonexistent subsequent turn – it often makes most sense strategically to play what I call the “sweatshop strategy”. The player switches off the automated production lines to save on the electricity bill, drags in all the seasonal workers they can muster, dusts off the old manpower-inefficient machines mouldering in the basement, and gets their army of workers cranking out widgets!
With indefinitely-increasing energy prices and functionally-flat staff costs, the rules of the game would always eventually reach the point at which it is most cost-effective
to switch to slave cheap labour rather than robots. but Factory Manager‘s fixed-duration means that this point often comes for all players in many games at the same
predictable point: a tipping point at which the free market backslides from automation to human labour to keep itself alive.
There are parallels in the real world. Earlier this month, Tim Watkins wrote:
The demise of the automated car wash may seem trivial next to these former triumphs of homo technologicus but it sits on the same continuum. It is just one of a gathering list of technologies that we used to be able to use, but can no longer express (through market or state spending) a purpose for. More worrying, however, is the direction in which we are willingly going in our collective decision to move from complexity to simplicity. The demise of the automated car wash has not followed a return to the practice of people washing their own cars (or paying the neighbours’ kid to do it). Instead we have more or less happily accepted serfdom (the use of debt and blackmail to force people to work) and slavery (the use of physical harm) as a reasonable means of keeping the cost of cleaning cars to a minimum (similar practices are also keeping the cost of food down in the UK). This, too, is precisely what is expected when the surplus energy available to us declines.
I love Factory Manager, but after reading Watkins’ article, it’ll probably feel a little different to play it, now. It’s like that moment when, while reading the rules, I first poured out the pieces of Puerto Rico. Looking through them, I thought for a moment about what the “colonist” pieces – little brown wooden circles brought to players’ plantations on ships in a volume commensurate with the commercial demand for manpower – represented. And that realisation adds an extra message to the game.
Beneath its (fabulous) gameplay, Factory Manager carries a deeper meaning encouraging the possibility of a discussion about capitalism, environmentalism, energy, and sustainability. And as our society falters in its ability to fulfil the techno-utopian dream, that’s perhaps a discussion we need to be having.
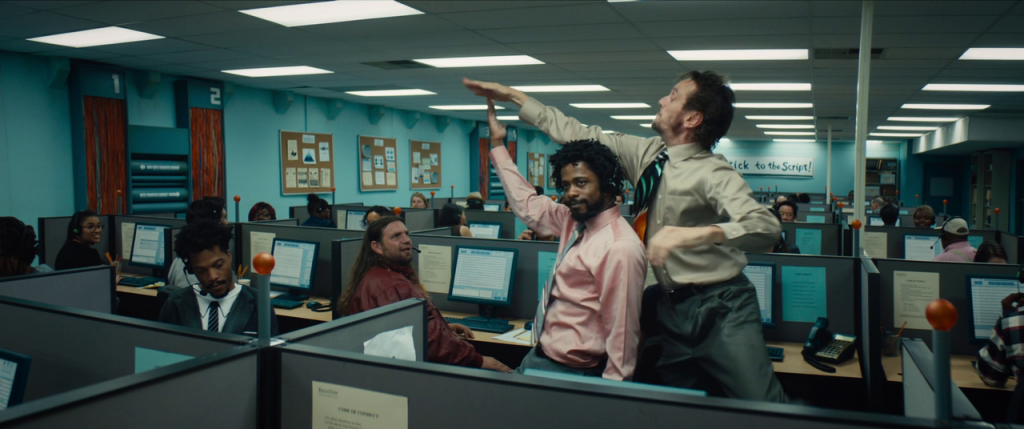
But for now, go watch Sorry to Bother You, where you’ll find further parallels… and at least you’ll get to laugh as you do so.
Note #12733
How To Play – Carcassonne
This article is a repost promoting content originally published elsewhere. See more things Dan's reposted.
This is not your typical How To Play. I’ll bet you never knew the real rules for Carcassonne…
REPORT: Cards Against Humanity officially surpasses acoustic guitars as the most annoying thing you can bring to a party – The Beaverton
This article is a repost promoting content originally published elsewhere. See more things Dan's reposted.
VICTORIA, BC – After data collection from thousands of parties across the country, reports are coming in that the annoying person who brings an acoustic guitar to a party is now officially less hated than the person who expects everyone to sit down and play Cards Against Humanity.
“At least when some asshole starts playing the tune to Wonderwall you can get up and go to a different room,” says Michelle Kalleta, 22, avid partygoer. “Jerks who show up with any sort of card game expect everyone to play, and the last thing I want to do at a party is sit in a circle with a bunch of people who think they’re hilariously edgy.”
…
Fnorders
I’ve not posted much recently: I’ve had a lot of Complicated Life Stuff going on, sorry.
But I did make a thing: fnorders.com. You’re welcome.